AlphaGo and AI Fears
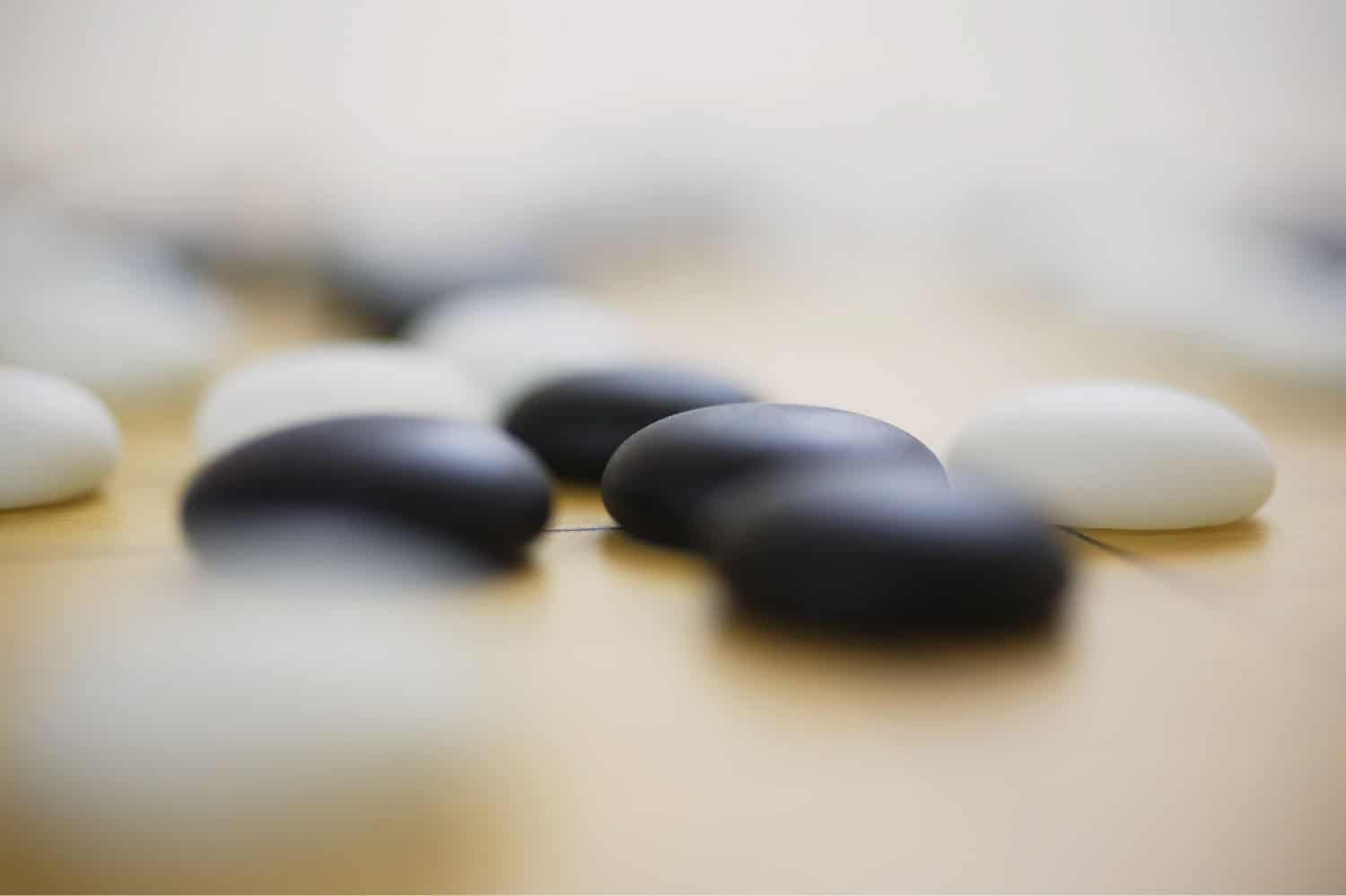
Contents
Two important pieces of AI news came out last week. The first, of course, was AlphaGo beating the world champion Go player, Lee Se-dol, 4-1 in their well publicized match up. The other was a survey by the British Science Association (BSA) that showed 60% of the public fear that AI will take over jobs, while 36% fear AI will destroy humanity.
The success of AlphaGo, though exciting and impressive, did not help to ease fears of an AI takeover. According to news reports, many in South Korea now fear artificial intelligence even more.
I spoke with a few people in AI, economics and anthropology to understand more about what the true implications of AlphaGo are and whether the public needs to be as worried as the BSA survey indicates.
AlphaGo is an interesting case because, as of a few months ago, most AI researchers would have said that a computer that could beat the world’s top humans at the game of Go was probably still a decade away. Yet, as AI expert Stuart Russell pointed out,
“From 10,000 feet there isn’t anything really radically new in the way that AlphaGo is designed.”
A variety of methods were employed by AlphaGo in order for it to learn to play Go as well as it does, but none of the methods stand out as new or even unique. They just hadn’t been put together like this before, and this level of computing power is greater than existed previously.
For example, the idea of using a minimax tree search, which essentially searches through all possible moves of a game (branches of the tree), was first considered for chess back in 1913. Evaluation functions were developed in the 1940s to take over when those tree searches got too big because too many moves were involved. Over the next twenty years, tree-pruning methods were developed and improved so programs could determine which sections of the tree represented moves that should be considered and which sections were unnecessary.
The 1940s also saw the development of neural nets, another common AI technique, which were inspired by biological neural architecture. In the 1950s, reinforcement learning was established, which maximizes rewards over time and creates an improvement loop for the evaluation function. By 1959, machine learning pioneer Aurther Samuel used these techniques to develop the first computer program that learned to play checkers.
Russell explained that the newest technique was that of roll-outs, which have greatly improved the tree search capabilities. Even this technique, though, has been around for at least a decade, if not a couple decades, depending on who one asks.
This combination of techniques allowed AlphaGo to learn the rules of Go and play against itself to continuously improve its strategy and ability.
If all of these techniques have been around for so long, why did AlphaGo come as such a surprise to the AI community?
Go is an incredibly large and complicated game, with more possible sequences of moves than there are atoms in the universe. According to Russell, the assumption until recently was that these more standard search and evaluation techniques wouldn’t be as effective for Go as they have been for chess. Researchers believed that in order for a computer program to successfully play Go, it would have to decompose the board game into parts, just as a human player does, and then pull each of those parts back together to analyze the quality of various moves. Research into Go AI hit a plateau for many years, and most AI experts expected that some new technique or capability would have to be developed in order for a program to perform this decomposition of possible outcomes.
Russell, in fact, had been hoping for such a decomposition result. This method would be much more consistent with how an AI would likely have to interact with the real world.
The success of AlphaGo may at least provide a deeper understanding of just how capable current AI methods and techniques are. In response to AlphaGo’s wins, Russell said,
“It tells you that the techniques may be more powerful than we thought.” He also added, “I’m glad it wasn’t 5-0.”
Victoria Krakovna, a co-founder of FLI and a machine learning researcher, was also impressed by the capabilities exhibited by these known programming techniques.
“To me,” Krakovna said, “this is another indication that AI is progressing faster than even many experts anticipated. The hybrid architecture of AlphaGo suggests that large advances can be made by combining existing components in the right way, in this case deep reinforcement learning and tree search. It’s possible that fewer novel breakthroughs remain than previously believed to reach general AI.”
But does this mean advanced AI is something we should fear?
While AI progress is something we want to move forward with in a safe and robust manner, AI researchers aren’t worried that the development of AlphaGo would soon lead to the destruction of humanity. The subject of jobs, though, is another story.
Even prominent AI researchers who aren’t worrying about AI as an existential risk, like Baidu’s Andrew Ng, are worried about the impact AI will have on the job market. However, that doesn’t mean all hope is lost.
In an email, Erik Brynjolfsson, an MIT economist and coauthor of The Second Machine Age, explained,
“Technology using AI will surely make it possible to eliminate some jobs but it will also make it possible to create new ones. The big question is whether the people who lost the old jobs will be able to do the new jobs. The answer will depend on our choices as individuals and as a society.”
Madeleine Clare Elish is a cultural anthropologist at Columbia University who focuses on the evolving role of humans in large-scale automated and autonomous systems. Her reaction to the BSA survey was similar to Brynjolfsson’s. She said:
“When the media focuses on AI in this way, it appears as if the technology develops on its own when, in fact, it is the product of human choices and values. And that is what we need to be talking about: what are the values that are driving AI innovation, and how can we make better choices that protect the public interest?
“When we rile up public fear about AI in the future, we miss all the things that are happening today. We need more people talking about the challenges facing the implementation of AI technologies, like preventing bias or other forms of unfairness in systems and issues of security and privacy.”
Both Brynjolfsson and Elish made a point of noting the choices we have before us. If we as a society can come together, even just a little, we can steer our future in a direction with a much more optimistic outlook for AI and humanity.
About the Future of Life Institute
The Future of Life Institute (FLI) is a global think tank with a team of 20+ full-time staff operating across the US and Europe. FLI has been working to steer the development of transformative technologies towards benefitting life and away from extreme large-scale risks since its founding in 2014. Find out more about our mission or explore our work.
Related content
Other posts about AI, Recent News
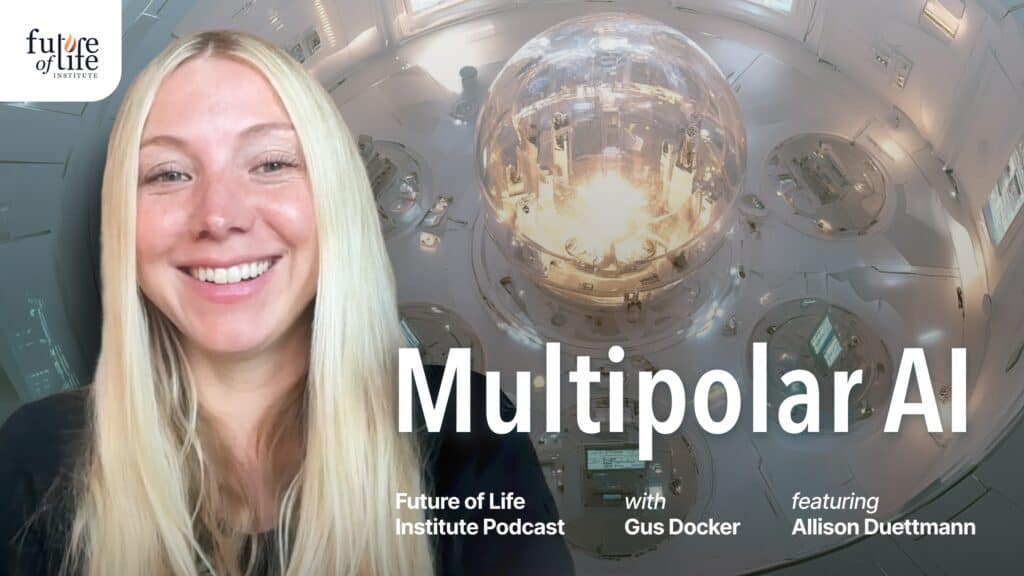
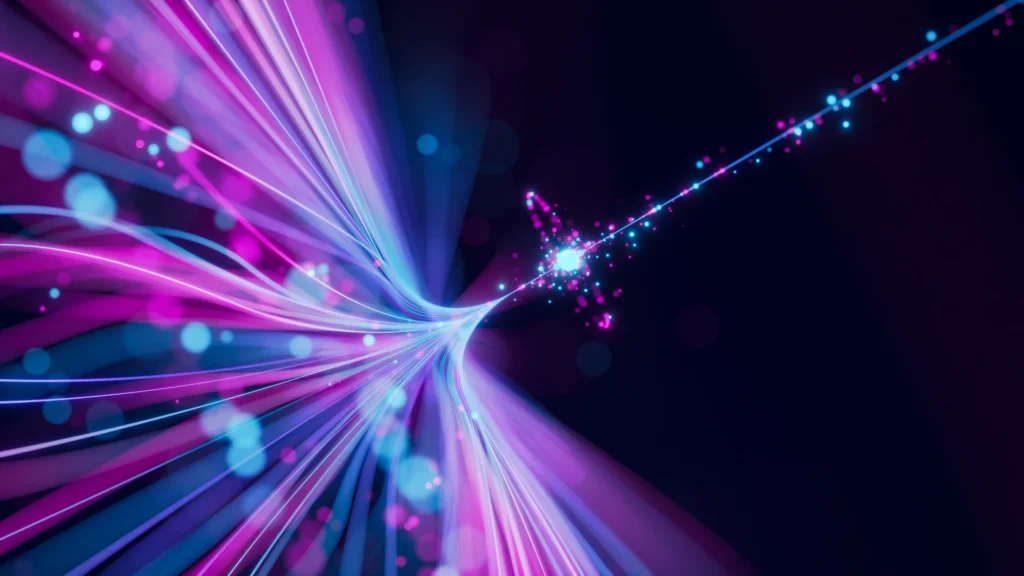