Eliezer Yudkowsky on AlphaGo’s Wins
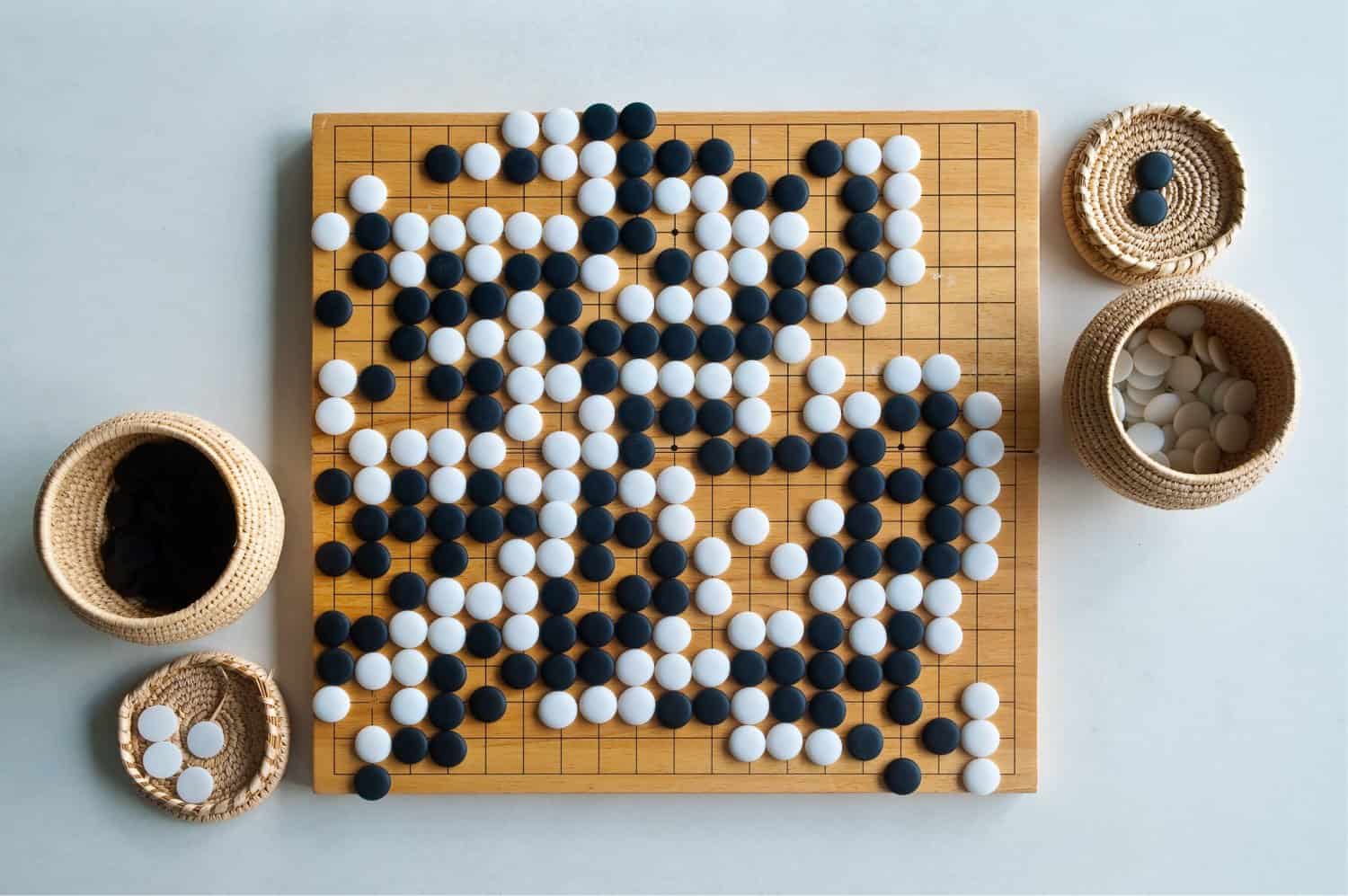
Contents
The following insightful article was written by Eliezer Yudkowsky and originally posted on his Facebook page.
(Note from the author about this post: Written after AlphaGo’s Game 2 win, posted as it was winning Game 3, and at least partially invalidated by the way AlphaGo lost Game 4. We may not know for several years what truly superhuman Go play against a human 9-pro looks like; and in particular, whether truly superhuman play shares the AlphaGo feature focused on here, of seeming to play games that look even to human players but that the machine player wins in the end. Some of the other points made do definitely go through for computer chess, in which unambiguously superhuman and non-buggy play has been around for years. Nonetheless, take with a grain of salt – I and a number of other people were surprised by AlphaGo’s loss in Game 4, and we don’t know as yet exactly how it happened and to what extent it’s a fundamental flaw versus a surface bug. What we might call the Kasparov Window – machine play that’s partially superhuman but still flawed enough for a human learner to understand, exploit, and sometimes defeat – may be generally wider than I thought.)
As I post this, AlphaGo seems almost sure to win the third game and the match.
At this point it seems likely that Sedol is actually far outclassed by a superhuman player. The suspicion is that since AlphaGo plays purely for probability of long-term victory rather than playing for points, the fight against Sedol generates boards that can falsely appear to a human to be balanced even as Sedol’s probability of victory diminishes. The 8p and 9p pros who analyzed games 1 and 2 and thought the flow of a seemingly Sedol-favoring game ‘eventually’ shifted to AlphaGo later, may simply have failed to read the board’s true state. The reality may be a slow, steady diminishment of Sedol’s win probability as the game goes on and Sedol makes subtly imperfect moves that humans think result in even-looking boards. (E.g., this analysis.)
For all we know from what we’ve seen, AlphaGo could win even if Sedol were allowed a one-stone handicap. But AlphaGo’s strength isn’t visible to us – because human pros don’t understand the meaning of AlphaGo’s moves; and because AlphaGo doesn’t care how many points it wins by, it just wants to be utterly certain of winning by at least 0.5 points.
IF that’s what was happening in those 3 games – and we’ll know for sure in a few years, when there’s multiple superhuman machine Go players to analyze the play – then the case of AlphaGo is a helpful concrete illustration of these concepts:
– Edge instantiation.
Extremely optimized strategies often look to us like ‘weird’ edges of the possibility space, and may throw away what we think of as ‘typical’ features of a solution. In many different kinds of optimization problem, the maximizing solution will lie at a vertex of the possibility space (a corner, an edge-case).
In the case of AlphaGo, an extremely optimized strategy seems to have thrown away the ‘typical’ production of a visible point lead that characterizes human play. Maximizing win-probability in Go, at this level of play against a human 9p, is not strongly correlated with what a human can see as visible extra territory – so that gets thrown out even though it was previously associated with ‘trying to win’ in human play.
– Unforeseen maximum.
Humans thought that a strong opponent would have more visible territory earlier – building up a lead seemed like an obvious way to ensure a win. But ‘gain more territory’ wasn’t explicitly encoded into AlphaGo’s utility function, and turned out not to be a feature of the maximum of AlphaGo’s actual utility function of ‘win the game’, contrary to human expectations of where that maximum would lie.
– Instrumental efficiency.
The human pros thought AlphaGo was making mistakes. Ha ha.
AlphaGo doesn’t actually play God’s Hand. Similarly, liquid stock prices sometimes make big moves. But human pros can’t detect AlphaGo’s departures from God’s Hand, and you can’t personally predict the net direction of stock price moves.
If you think the best move is X and AlphaGo plays Y, we conclude that X had lower expected winningness than you thought, or that Y had higher expected winningness than you thought. We don’t conclude that AlphaGo made an inferior move.
Thinking you can spot AlphaGo’s mistakes is like thinking your naked eye can see an exploitable pattern in S&P 500 price moves – we start out with a very strong suspicion that you’re mistaken, overriding the surface appearance of reasonable arguments.
– Convergence to apparent consequentialism / explanation by final causes.
Early chess-playing programs would do things that humans could interpret in terms of the chess-playing program having particular preferences or weaknesses, like “The program doesn’t understand center strategy very well” or (much earlier) “The program has a tendency to move its queen a lot.”
This ability to explain computer moves in ‘psychological’ terms vanished as computer chess improved. For a human master looking at a modern chess program, their immediate probability distribution on what move the chess algorithm outputs, should be the same as their probability distribution on the question “Which move will in fact lead to a future win?” That is, if there were a time machine that checked the (conditional) future and output a move such that it would in fact lead to a win for the chess program, then your probability distribution on the time machine’s next immediate move, and your probability distribution on the chess program’s next immediate move, would be the same.
Of course chess programs aren’t actually as powerful as time machines that output a Path to Victory; the actual moves output aren’t the same. But from a human perspective, there’s no difference in how we predict the next move, at least if we have to do it using our own intelligence without computer help. At this point in computer chess, a human might as well give up on every part of the psychological explanation for any move a chess program makes, like “It has trouble understanding the center” or “It likes moving its queen”, leaving only, “It output that move because that is the move that leads to a future win.”
This is particularly striking in the case of AlphaGo because of the stark degree to which “AlphaGo output that move because the board will later be in a winning state” sometimes doesn’t correlate with conventional Go goals like taking territory or being up on points. The meaning of AlphaGo’s moves – at least some of the moves – often only becomes apparent later in the game. We can best understand AlphaGo’s output in terms of the later futures to which it leads, treating it like a time machine that follows a Path to Victory.
Of course, in real life, there’s a way AlphaGo’s move was computed and teleological retrocausation was not involved. But you can’t relate the style of AlphaGo’s computation to the style of AlphaGo’s move in any way that systematically departs from just reiterating “that output happened because it will lead to a winning board later”. If you could forecast a systematic departure between what those two explanations predict in terms of immediate next moves, you would know an instrumental inefficiency in AlphaGo.
This is why the best way to think about a smart paperclip maximizer is to imagine a time machine whose output always happens to lead to the greatest number of paperclips. A real-world paperclip maximizer wouldn’t actually have that exactly optimal output, and you can expect that in the long run the real-world paperclip maximizer will get less paperclips than an actual time machine would get. But you can never forecast a systematic difference between your model of the paperclip maximizer’s strategy, and what you imagine the time machine would do – that’s postulating a publicly knowable instrumental inefficiency. So if we’re trying to imagine whether a smart paperclip maximizer would do X, we ask “Does X lead to the greatest possible number of expected paperclips, without there being any alternative Y that leads to more paperclips?” rather than imagining the paperclip maximizer as having a psychology.
And even then your expectation of the paperclip maximizer actually doing X should be no stronger than your belief that you can forecast AlphaGo’s exact next move, which by Vingean uncertainty cannot be very high. If you knew exactly where AlphaGo would move, you’d be that smart yourself. You should, however, expect the paperclip maximizer to get at least as many paperclips as you think could be gained from X, unless there’s some unknown-to-you flaw in X and there’s no better alternative.
– Cognitive uncontainability.
Human pros can’t predict where AlphaGo will move because AlphaGo searches more possibilities than human pros have time to consider. It’s not just that AlphaGo estimates value differently, but that the solution AlphaGo finds that maximizes AlphaGo’s estimated value, is often outside the set of moves whose value you were calculating.
– Strong cognitive uncontainability.
Even after the human pros saw AlphaGo’s exact moves, the humans couldn’t see those moves as powerful strategies, not in advance and sometimes not even after the fact, because the humans lacked the knowledge to forecast the move’s consequences.
Imagine someone in the 11th century trying to figure out how people in the 21st century might cool their houses. Suppose that they had enough computing power to search lots and lots of possible proposals, but had to use only their own 11th-century knowledge of how the universe worked to evaluate those proposals. Suppose they had so much computing power that at some point they randomly considered a proposal to construct an air conditioner. If instead they considered routing water through a home and evaporating the water, that might strike them as something that could possibly make the house cooler, if they saw the analogy to sweat. But if they randomly consider the mechanical diagram of an air conditioner as a possible solution, they’ll toss it off as a randomly generated arcane diagram. They can’t understand why this would be an effective strategy for cooling their house, because they don’t know enough about thermodynamics and the pressure-heat relation.
The gap between the 11th century and the 21st century isn’t just the computing power to consider more alternatives. Even if the 11th century saw the solutions we used, they wouldn’t understand why they’d work – lacking other reasons to trust us, they’d look at the air conditioner diagram and say “Well that looks stupid.”
Similarly, it’s not just that humans lack the computing power to search as many moves as AlphaGo, but that even after AlphaGo plays the move, we don’t understand its consequences. Sometimes later in the game we see the consequences of a good move earlier, but that’s only one possible way that Sedol played out the game, so we don’t understand the value of many other moves. We don’t realize how much expected utility is available to AlphaGo, not just because AlphaGo searches a wider space of possibilities, but because we lack the knowledge needed to understand what AlphaGo’s moves will do.
This is the kind of cognitive uncontainability that would apply if the 11th century was trying to forecast how much cooling would be produced by the best 21st-century solution for cooling a house. From an 11th-century perspective, the 21st century has ‘magic’ solutions that do better than their best imaginable solutions and that they wouldn’t understand even if they had enough computing power to consider them as possible actions.
Go is a domain much less rich than the real world, and it has rigid laws we understand in toto. So superhuman Go moves don’t contain the same level of sheer, qualitative magic that the 21st century has from the perspective of the 19th century. But Go is rich enough to demonstrate strong cognitive uncontainability on a small scale. In a rich and complicated domain whose rules aren’t fully known, we should expect even more magic from superhuman reasoning – solutions that are better than the best solution we could imagine, operating by causal pathways we wouldn’t be able to foresee even if we were told the AI’s exact actions.
For an example of an ultra-complicated poorly understood domain where we should reasonably expect that a smarter intelligence can deploy ‘magic’ in this sense, consider, say, the brain of a human gatekeeper trying to keep an AI in a box. Brains are very complicated, and we don’t understand them very well. So superhuman moves on that gameboard will look to us like magic to a much greater extent than AlphaGo’s superhuman Go moves.
– Patience.
A paperclip maximizer doesn’t bother to make paperclips until it’s finished doing all the technology research and has gained control of all matter in its vicinity, and only then does it switch to an exploitation strategy. Similarly, AlphaGo has no need to be “up on (visible) points” early. It simply sets up the thing it wants, win probability, to be gained at the time it wants it.
– Context change and sudden turns.
By sheer accident of the structure of Go and the way human 9ps play against superior opponents – namely, giving away probability margins they don’t understand while preserving their apparent territory – we’ve ended up with an AI that is apparently not being superhumanly dangerous until, you know, it just happens to win at the end.
Now in this case, that’s happening because of a coincidence of the game structure, not because AlphaGo models human minds and hides how far it’s ahead. I mean, maybe DeepMind deliberately built this version of AlphaGo to exploit human opponents, or a similar pattern emerged from trial-and-error uncovering systems that fought particularly well against human players. But if the architecture is still basically like the October AlphaGo architecture, which seems more probable, then AlphaGo acts as if it’s playing another AlphaGo; that’s how all of the internal training worked and how all of its future forecasts worked in the October version. AlphaGo probably has no model of humans and no comprehension that this time it’s fighting Sedol instead of another computer. So AlphaGo’s underplayed strength isn’t deliberate… probably.
So this is not the same phenomenon as the expected convergent incentive, following a sufficiently cognitively powerful AI noticing a divergence between what it wants and what the programmers want, for that AI to deceive the programmers about how smart it is. Or the convergent instrumental incentive for that AI to not strike out, or even give any sign whatsoever that anything is wrong, until it’s ready to win with near certainty.
But AlphaGo is still a nice accidental illustration that when you’ve been placed in an adversarial relation to something smarter than you, you don’t always know that you’ve lost, or that anything is even wrong, until the end.
– Rapid capability gain and upward-breaking curves.
“Oh, look,” I tweeted, “it only took 5 months to go from landing one person on Mars to Mars being overpopulated.” (In reference to Andrew Ng’s claim that worrying about AGI outcomes is like worrying about overpopulation on Mars.)
The case of AlphaGo serves as a possible rough illustration of what might happen later. Later on, there’s an exciting result in a more interesting algorithm that operates on a more general level (I’m not being very specific here, for the same reason I don’t talk about my ideas for building really great bioweapons). The company dumps in a ton of research effort and computing power. 5 months later, a more interesting outcome occurs.
Martian population growth doesn’t always work on smooth, predictable curves that everyone can see coming in advance. The more powerful the AI technology, the more it makes big jumps driven by big insights. As hardware progress goes on, those big insights can be applied over more existing hardware to produce bigger impacts. We’re not even in the recursive regime yet, and we’re still starting to enter the jumpy unpredictable phase where people are like “What just happened?”
– Local capability gain.
So far as I can tell, if you look at everything that Robin Hanson said about distributed FOOM and everything I said about local FOOM in the Hanson-Yudkowsky FOOM debate, everything about AlphaGo worked out in a way that matches the “local” model of how things go.
One company with a big insight jumped way ahead of everyone else. This is true even though, since the world wasn’t at stake this time, DeepMind actually published their recipe for the October version of their AI.
AlphaGo’s core is built around a similar machine learning technology to DeepMind’s Atari-playing system – the single, untweaked program that was able to learn superhuman play on dozens of different Atari games just by looking at the pixels, without specialization for each particular game. In the Atari case, we didn’t see a bunch of different companies producing gameplayers for all the different varieties of game. The Atari case was an example of an event that Robin Hanson called “architecture” and doubted, and that I called “insight.” Because of their big architectural insight, DeepMind didn’t need to bring in lots of different human experts at all the different Atari games to train their universal Atari player. DeepMind just tossed all pre-existing expertise because it wasn’t formatted in a way their insightful AI system could absorb, and besides, it was a lot easier to just recreate all the expertise from scratch using their universal Atari-learning architecture.
The October version of AlphaGo did initially seed one of the key components by training it to predict a big human database of games. But Demis Hassabis has suggested that next up after this competition will be getting DeepMind to train itself in Go entirely from scratch, tossing the 2500-year human tradition right out the window.
More importantly, so far as I know, AlphaGo wasn’t built in collaboration with any of the commercial companies that built their own Go-playing programs for sale. The October architecture was simple and, so far as I know, incorporated very little in the way of all the particular tweaks that had built up the power of the best open-source Go programs of the time. Judging by the October architecture, after their big architectural insight, DeepMind mostly started over in the details (though they did reuse the widely known core insight of Monte Carlo Tree Search). DeepMind didn’t need to trade with any other Go companies or be part of an economy that traded polished cognitive modules, because DeepMind’s big insight let them leapfrog over all the detail work of their competitors.
Frankly, this is just how things have always worked in the AI field and I’m not sure anyone except Hanson expects this to change. But it’s worth noting because Hanson’s original reply, when I pointed out that no modern AI companies were trading modules as of 2008, was “That’s because current AIs are terrible and we’ll see that changing as AI technology improves.” DeepMind’s current AI technology is less terrible. The relevant dynamics haven’t changed at all. This is worth observing.
– Human-equivalent competence is a small and undistinguished region in possibility-space.
As I tweeted early on when the first game still seemed in doubt, “Thing that would surprise me most about ‪#‎alphago‬ vs. ‪#‎sedol‬: for either player to win by three games instead of four or five.”
Since DeepMind picked a particular challenge time in advance, rather than challenging at a point where their AI seemed just barely good enough, it was improbable that they’d make exactly enough progress to give Sedol a nearly even fight.
AI is either overwhelmingly stupider or overwhelmingly smarter than you. The more other AI progress and the greater the hardware overhang, the less time you spend in the narrow space between these regions. There was a time when AIs were roughly as good as the best human Go-players, and it was a week in late January.
: AlphaGo’s strange, losing play in the 4th game suggests that playing seemingly-near-even games might possibly be a ‘psychological’ feature of the Monte Carlo algorithm rather than alien-efficient play. But again, we’ll know for sure in a few years when there’s debugged, unambiguously superhuman machine Go players.
That doesn’t mean AlphaGo is only slightly above Lee Sedol, though. It probably means it’s “superhuman with bugs”. That’s one of the Interesting scenarios that MIRI hasn’t been trying to think through in much detail, because (1) it’s highly implementation-dependent and I haven’t thought of anything general to say, and (2) it only shows up in AGI scenarios with limited or no AI self-improvement, and we’re only just starting to turn our attention to those. As it stands, it seems AlphaGo plays a mix of mostly ‘stupid’ moves that are too smart for humans to comprehend, plus a few ‘stupid’ moves that are actually stupid. Let’s try to avoid this scenario in real-world AGI.
Note that machine chess has been out of the flawed superhuman regime and well into the pure superhuman regime for years now. So everything above about instrumental efficiency goes through for machine chess without amendment – we say ‘ha ha’ to any suggestion that the smartest human can see a flaw in play with their naked eye, if you think X is best and the machine player does Y then we conclude you were wrong, and so on.
About the Future of Life Institute
The Future of Life Institute (FLI) is a global think tank with a team of 20+ full-time staff operating across the US and Europe. FLI has been working to steer the development of transformative technologies towards benefitting life and away from extreme large-scale risks since its founding in 2014. Find out more about our mission or explore our work.
Related content
Other posts about AI, Recent News
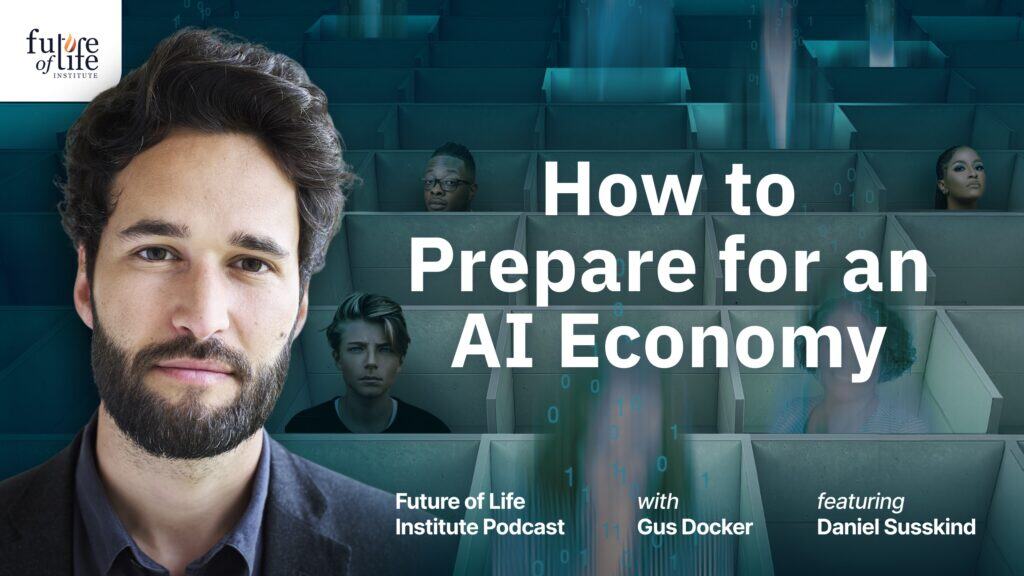