Introductory Resources on AI Safety Research
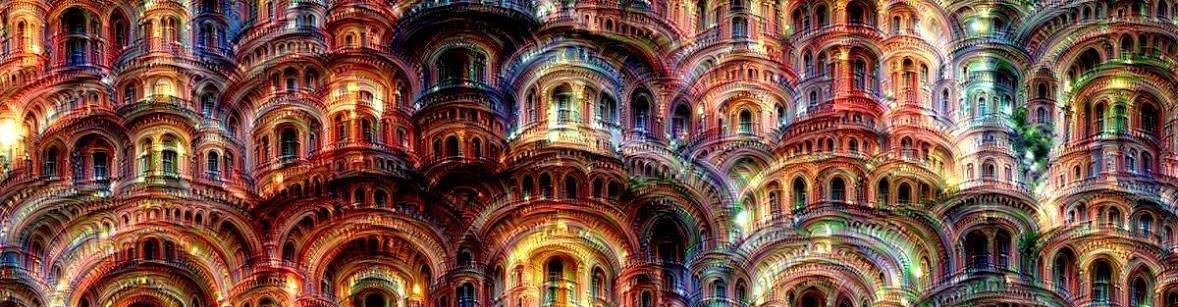
Contents
Reading list to get up to speed on the main ideas in the field. The resources are selected for relevance and/or brevity, and the list is not meant to be comprehensive.
Motivation
For a popular audience:
Cade Metz, 2017. New York Times: Teaching A.I. Systems to Behave Themselves
FLI. AI risk background and FAQ. At the bottom of the background page, there is a more extensive list of resources on AI safety.
Tim Urban, 2015. Wait But Why: The AI Revolution. An accessible introduction to AI risk forecasts and arguments (with cute hand-drawn diagrams, and a few corrections from Luke Muehlhauser).
OpenPhil, 2015. Potential risks from advanced artificial intelligence. An overview of AI risks and timelines, possible interventions, and current actors in this space.
For a more technical audience:
Stuart Russell:
- The long-term future of AI (longer version), 2015. A video of Russell’s classic talk, discussing why it makes sense for AI researchers to think about AI safety, and going over various misconceptions about the issues.
- Concerns of an AI pioneer, 2015. An interview with Russell on the importance of provably aligning AI with human values, and the challenges of value alignment research.
- On Myths and Moonshine, 2014. Russell’s response to the “Myth of AI” question on Edge.org, which draws an analogy between AI research and nuclear research, and points out some dangers of optimizing a misspecified utility function.
Scott Alexander, 2015. No time like the present for AI safety work. An overview of long-term AI safety challenges, e.g. preventing wireheading and formalizing ethics.
Victoria Krakovna, 2015. AI risk without an intelligence explosion. An overview of long-term AI risks besides the (overemphasized) intelligence explosion / hard takeoff scenario, arguing why intelligence explosion skeptics should still think about AI safety.
Stuart Armstrong, 2014. Smarter Than Us: The Rise Of Machine Intelligence. A short ebook discussing potential promises and challenges presented by advanced AI, and the interdisciplinary problems that need to be solved on the way there.
Technical overviews
Soares and Fallenstein, 2017. Aligning Superintelligence with Human Interests: A Technical Research Agenda
Amodei, Olah, et al, 2016. Concrete Problems in AI safety. Research agenda focusing on accident risks that apply to current ML systems as well as more advanced future AI systems.
Jessica Taylor et al, 2016. Alignment for Advanced Machine Learning Systems
FLI, 2015. A survey of research priorities for robust and beneficial AI
Jacob Steinhardt, 2015. Long-Term and Short-Term Challenges to Ensuring the Safety of AI Systems. A taxonomy of AI safety issues that require ordinary vs extraordinary engineering to address.
Nate Soares, 2015. Safety engineering, target selection, and alignment theory. Identifies and motivates three major areas of AI safety research.
Nick Bostrom, 2014. Superintelligence: Paths, Dangers, Strategies. A seminal book outlining long-term AI risk considerations.
Steve Omohundro, 2007. The basic AI drives. A classic paper arguing that sufficiently advanced AI systems are likely to develop drives such as self-preservation and resource acquisition independently of their assigned objectives.
Technical work
Value learning:
Smitha Milli et al. Should robots be obedient? Obedience to humans may sound like a great thing, but blind obedience can get in the way of learning human preferences.
William Saunders et al, 2017. Trial without Error: Towards Safe Reinforcement Learning via Human Intervention. (blog post)
Amin, Jiang, and Singh, 2017. Repeated Inverse Reinforcement Learning. Separates the reward function into a task-specific component and an intrinsic component. In a sequence of task, the agent learns the intrinsic component while trying to avoid surprising the human.
Dylan Hadfield-Menell et al, 2016. Cooperative inverse reinforcement learning. Defines value learning as a cooperative game where the human tries to teach the agent about their reward function, rather than giving optimal demonstrations like in standard IRL.
Owain Evans et al, 2016. Learning the Preferences of Ignorant, Inconsistent Agents.
Reward gaming / wireheading:
Tom Everitt et al, 2017. Reinforcement learning with a corrupted reward channel. A formalization of the reward misspecification problem in terms of true and corrupt reward, a proof that RL agents cannot overcome reward corruption, and a framework for giving the agent extra information to overcome reward corruption. (blog post)
Amodei and Clark, 2016. Faulty Reward Functions in the Wild. An example of reward function gaming in a boat racing game, where the agent gets a higher score by going in circles and hitting the same targets than by actually playing the game.
Everitt and Hutter, 2016. Avoiding Wireheading with Value Reinforcement Learning. An alternative to RL that reduces the incentive to wirehead.
Laurent Orseau, 2015. Wireheading. An investigation into how different types of artificial agents respond to opportunities to wirehead (unintended shortcuts to maximize their objective function).
Interruptibility / corrigibility:
Dylan Hadfield-Menell et al. The Off-Switch Game. This paper studies the interruptibility problem as a game between human and robot, and investigates which incentives the robot could have to allow itself to be switched off.
El Mahdi El Mhamdi et al, 2017. Dynamic Safe Interruptibility for Decentralized Multi-Agent Reinforcement Learning.
Orseau and Armstrong, 2016. Safely interruptible agents. Provides a formal definition of safe interruptibility and shows that off-policy RL agents are more interruptible than on-policy agents. (blog post)
Nate Soares et al, 2015. Corrigibility. Designing AI systems without incentives to resist corrective modifications by their creators.
Scalable oversight:
Christiano, Leike et al, 2017. Deep reinforcement learning from human preferences. Communicating complex goals to AI systems using human feedback (comparing pairs of agent trajectory segments).
David Abel et al. Agent-Agnostic Human-in-the-Loop Reinforcement Learning.
Other:
Armstrong and Levinstein, 2017. Low Impact Artificial Intelligences. An intractable but enlightening definition of low impact for AI systems.
Babcock, Kramar and Yampolskiy, 2017. Guidelines for Artificial Intelligence Containment.
Scott Garrabrant et al, 2016. Logical Induction. A computable algorithm for the logical induction problem.
Note: I did not include literature on less neglected areas of the field like safe exploration, distributional shift, adversarial examples, or interpretability (see e.g. Concrete Problems or the CHAI bibliography for extensive references on these topics).
Collections of technical works
FLI grantee publications (scroll down)
Paul Christiano. AI control. A blog on designing safe, efficient AI systems (approval-directed agents, aligned reinforcement learning agents, etc).
If there are any resources missing from this list that you think are a must-read, please let me know! If you want to go into AI safety research, check out these guidelines and the AI Safety Syllabus.
(Thanks to Ben Sancetta, Taymon Beal and Janos Kramar for their feedback on this post.)
This article was originally posted on Victoria Krakovna’s blog.
About the Future of Life Institute
The Future of Life Institute (FLI) is a global non-profit with a team of 20+ full-time staff operating across the US and Europe. FLI has been working to steer the development of transformative technologies towards benefitting life and away from extreme large-scale risks since its founding in 2014. Find out more about our mission or explore our work.
Related content
Other posts about AI, Recent News
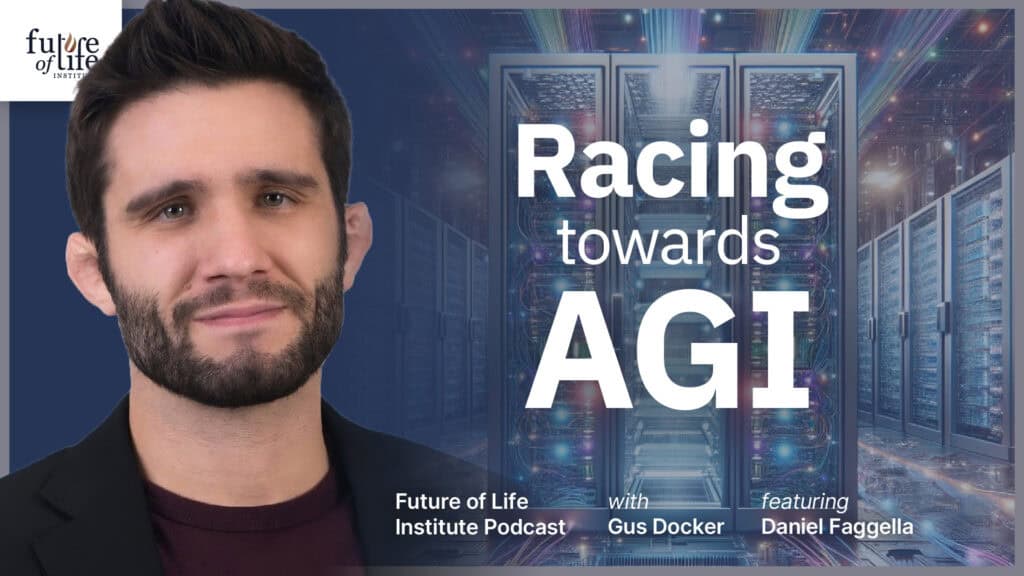
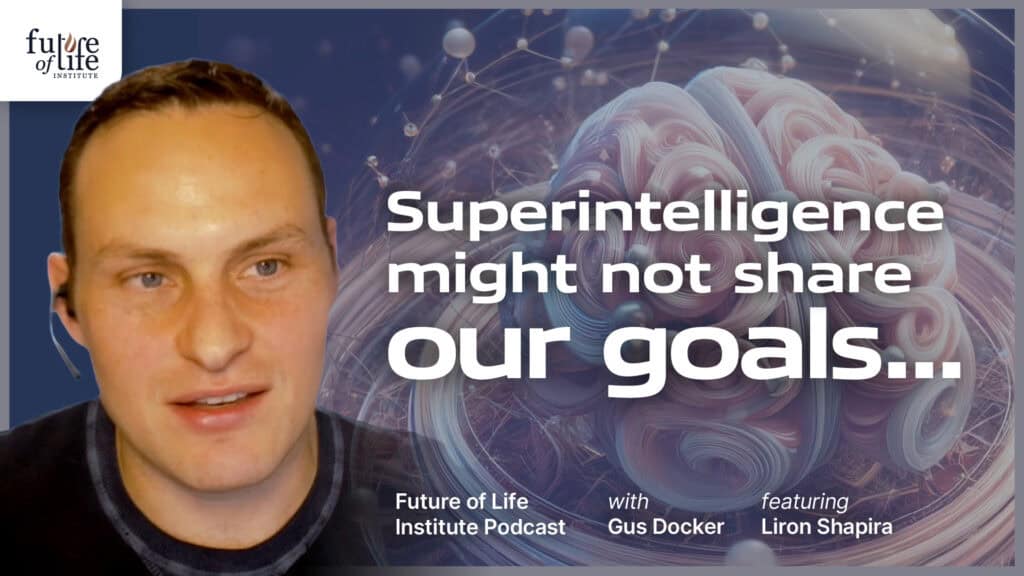
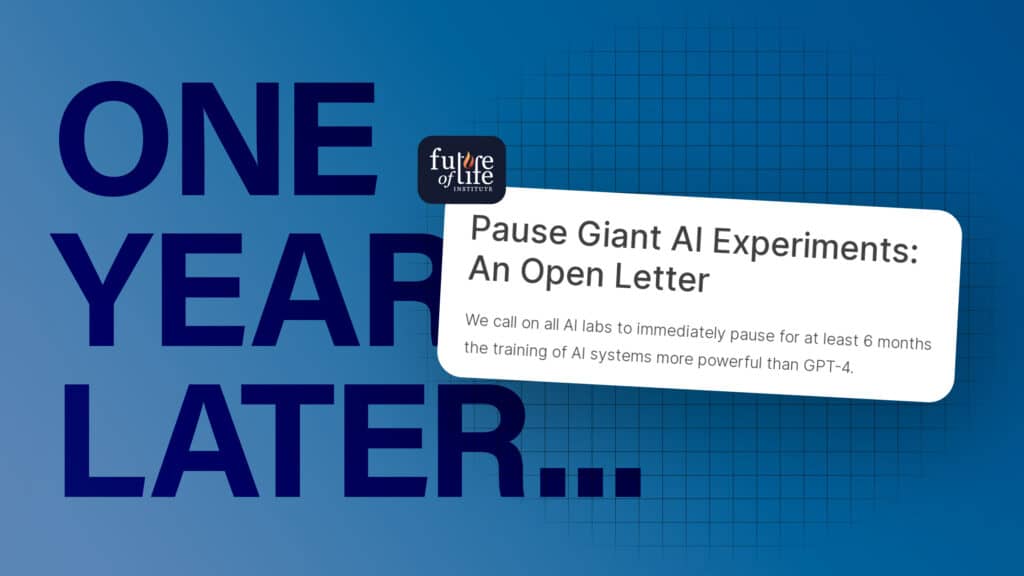
The Pause Letter: One year later
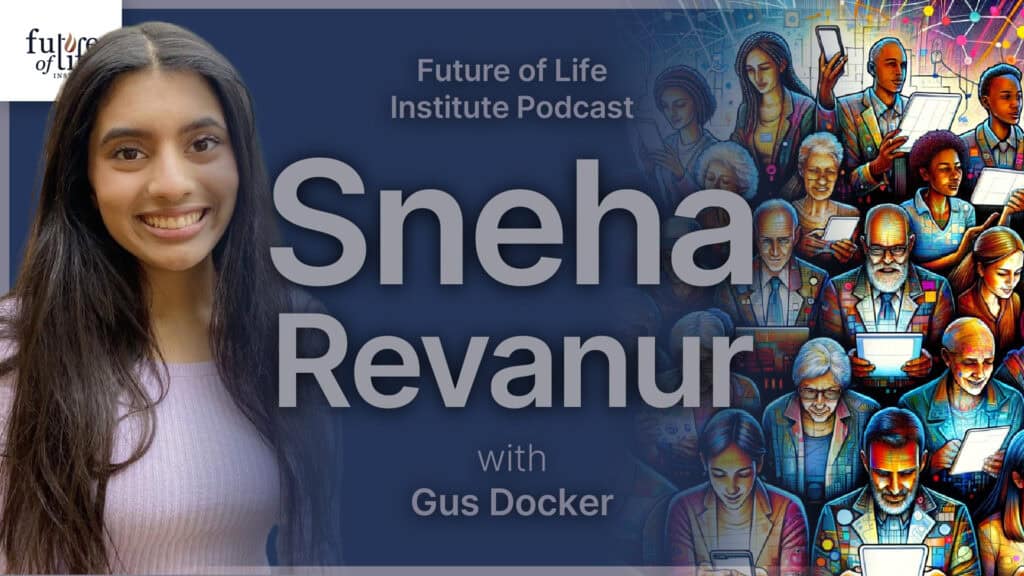