FLI Podcast: Applying AI Safety & Ethics Today with Ashley Llorens & Francesca Rossi
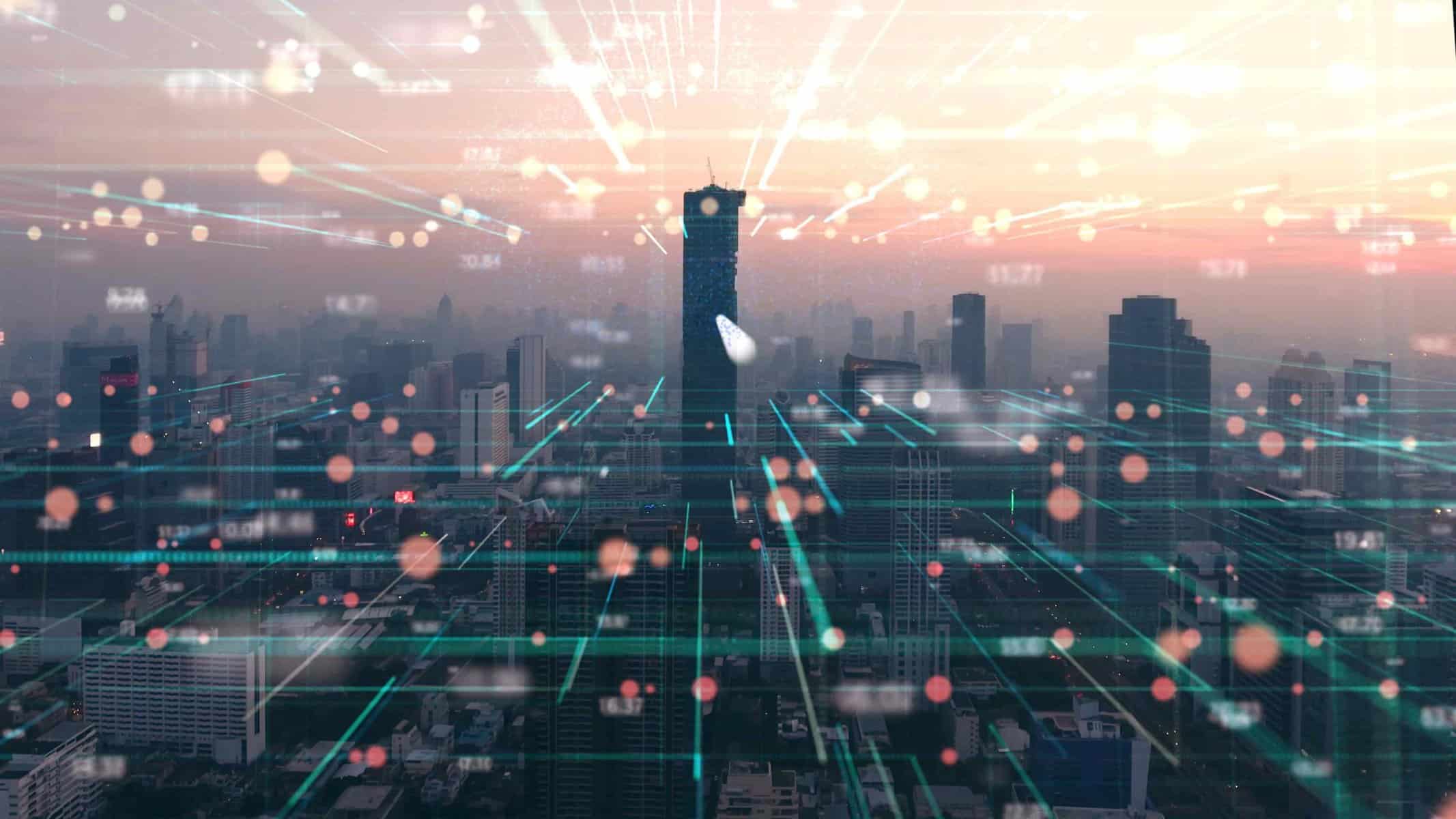
As we grapple with questions about AI safety and ethics, we're implicitly asking something else: what type of a future do we want, and how can AI help us get there?
In this month’s podcast, Ariel spoke with Ashley Llorens, the Founding Chief of the Intelligent Systems Center at the Johns Hopkins Applied Physics Laboratory, and Francesca Rossi, the IBM AI Ethics Global Leader at the IBM TJ Watson Research Lab and an FLI board member, about developing AI that will make us safer, more productive, and more creative. Too often, Rossi points out, we build our visions of the future around our current technology. Here, Llorens and Rossi take the opposite approach: let's build our technology around our visions for the future.
Topics discussed in this episode include:
- Hopes for the future of AI
- AI-human collaboration
- AI’s influence on art and creativity
- The UN AI for Good Summit
- Gaps in AI safety
- Preparing AI for uncertainty
- Holding AI accountable
Publications and resources discussed in this episode include:
- UN Sustainable Development Goals
- Everyday Ethics for Artificial Intelligence: A Practical Guide for Designers and Developers
Transcript
Ariel: Hello and welcome to another episode of the FLI podcast. I'm your host Ariel Conn, and today we'll be looking at how to address safety and ethical issues surrounding artificial intelligence, and how we can implement safe and ethical AIs both now and into the future. Joining us this month are Ashley Llorens and Francesca Rossi who will talk about what they're seeing in academia, industry, and the military in terms of how AI safety is already being applied and where the gaps are that still need to be addressed.
Ashley is the Founding Chief of the Intelligent Systems Center at the John Hopkins Applied Physics Laboratory where he directs research and development in machine learning, robotics, autonomous systems, and neuroscience all towards addressing national and global challenges. He has served on the Defense Science Board, the Naval Studies Board of the National Academy of Sciences, and the Center for a New American Security's AI task force. He is also a voting member of the Recording Academy, which is the organization that hosts the Grammy Awards, and I will definitely be asking him about that later in the show.
Francesca is the IBM AI Ethics Global Leader at the IBM TJ Watson Research Lab. She is an advisory board member for FLI, a founding board member for the Partnership on AI, a deputy academic director of the Leverhulme Centre for the Future of Intelligence, a fellow with AAAI and EurAI (that’s e-u-r-a-i), and she will be the general chair of AAAI in 2020. She was previously Professor of Computer Science at the University of Padova in Italy, and she's been president of IJCAI and the editor-in-chief of the Journal of AI Research. She is currently joining us from the United Nations AI For Good Summit, which I will also ask about later in the show.
So Ashley and Francesca, thank you so much for joining us today.
Francesca: Thank you.
Ashley: Glad to be here.
Ariel: Alright. The first question that I have for both of you, and Ashley, maybe I'll direct this towards you first: basically, as you look into the future and you look at artificial intelligence becoming more of a role in our everyday lives — before we look at how everything could go wrong, what are we striving for? What do you hope will happen with artificial intelligence and humanity?
Ashley: My perspective on AI is informed a lot by my research and experiences at the Johns Hopkins Applied Physics Lab, which I've been at for a number of years. My earliest explorations had to do with applications of artificial intelligence to robotics systems, in particular underwater robotics systems, systems where signal processing and machine learning are needed to give the system situational awareness. And of course, light doesn't travel very well underwater, so it's an interesting task to make a machine see with sound for all of its awareness and all of its perception.
And in that journey, I realized how hard it is to have AI-enabled systems capable of functioning in the real world. That's really been a personal research journey that's turned into an institution-wide research journey for Johns Hopkins APL writ large. And we're a large not-for-profit R & D organization that does national security, space exploration, and health. We're about 7,000 folks or so across many different disciplines, but many scientists and engineers working on those kinds of problems — we say critical contributions to critical challenges.
So as I look forward, I'm really looking at AI-enabled systems, whether they're algorithmic in cyberspace or they're real-world systems that are really able to act with greater autonomy in the context of these important national and global challenges. So for national security: to have robotic systems that can be where people don't want to be, in terms of being under the sea or even having a robot go into a situation that could be dangerous so a person doesn't have to. And to have that system be able to deal with all the uncertainty associated with that.
You look at future space exploration missions where — in terms of AI for scientific discovery, we talk a lot about that — imagine a system that can perform science with greater degrees of autonomy and figure out novel ways of using its instruments to form and interrogate hypotheses when billions of miles away. Or in health applications where we can have systems more ubiquitously interpreting data and helping us to make decisions about our health to increase our lifespan, or health span as they say.
I've been accused of being a techno-optimist, I guess. I don't think technology is the solution to everything, but it is my personal fascination. And in general, just having this AI capable of adding value for humanity in a real world that's messy and sloppy and uncertain.
Ariel: Alright. Francesca, you and I have talked a bit in the past, and so I know you do a lot of work with AI safety and ethics. But I know you're also incredibly hopeful about where we can go with AI. So if you could start by talking about some of the things that you're most looking forward to.
Francesca: Sure. Partially focused on the need of developing autonomous AI systems that can act where humans cannot go, for example, and that's definitely very, very important. I would like to focus more on the need also of AI systems that can actually work together with humans, augmenting our own capabilities to make decisions or to function in our work environment or in our private environment. That's the focus of and the purpose of AI that I see, that I work on, and I focus on what are the challenges in making this system really work well with humans.
This means of course that while it may seem that in some sense it's easier to develop an AI system that works together with humans because there is complementarity — some things are made by humans, some things are made by the machine. But actually, there are several additional challenges because you want these two entities, the human and the machine, to actually become a real team and work together and collaborate together to achieve a certain goal. You want these machines to be able to communicate, interact in a very natural way with human beings and you want these machines to be not just reactive to commands, but also proactive at trying to understand what the human being needs in that moment, in that context in order to provide all the information and knowledge that it needs from the data that surrounds whatever task is going to be addressed.
That's the focus also of what IBM Business Model is, because of course IBM releases AI to be used in other companies so that their professional people can use it to do better the job that they're doing. And it has many, many different interesting research directions. The one that I'm mostly focused on is around value alignment. How do you make sure that these systems know and are aware of the values that they should follow and of the ethical principles that they should follow, while trying to help human beings do whatever they need to do? And there are many ways that you can do that and many ways to model them to reason with these ethical principles and so on.
Being here in Geneva at AI For Good, I mean, in general, I think that here for example the emphasis is — and rightly so — about the sustainable development goals of the UN: these 17 goals that define a vision of the future, the future that we want. And we're trying to understand how we can leverage technologies such as AI to achieve that vision. The vision can be slightly nuanced and different, but to me, the development of advanced AI is not the end goal, but is only a way to get to the vision of the future that I have. And so, to me, this AI For Good Summit and the 17 sustainable development goals define a vision of the future that is important to have when one has in mind how to improve technology.
Ariel: For listeners who aren't as familiar with the sustainable development goals, we can include links to what all of those are in the podcast description.
Francesca: I was impressed at this AI For Good Summit. This Summit started three years ago with kind of 400 people. Then last year it was like 500 people, and this year there are 3,200 registered participants. To really give you an idea of how more and more everybody's interested into these subjects.
Ariel: Have you also been equally impressed by the topics that are covered?
Francesca: Well, I mean, it started today. So I just saw in the morning there are five different parallel sessions that will go throughout the following two days. One is AI education and learning. One is health and wellbeing. One is AI human dignity and inclusive society. One is scaling AI for good. And one is AI for space. These five themes will go throughout two days together with many other smaller ones. But for what I've seen this morning, it's really a very high level of the discussion. It's going to be very impactful. Each event is unique, has its own specificity, but this event is unique because it's focused on a vision of the future, which in this case are the sustainable development goals.
Ariel: Well, I'm really glad that you're there. We're excited to have you there. And so, you're talking about moving towards futures where we have AIs that can do things that either humans can't do or don't want to do or isn't safe, visions where we can achieve more because we're working with AI systems as opposed to just humans trying to do things alone. But we still have to get to those points where this is being implemented safely and ethically.
I'll come back to the question of what we're doing right so far, but first, what do you see as the biggest gaps in AI safety and ethics? And this is a super broad question, but looking at it with respect to, say, the military or industry or academia. What are some of the biggest problems you see in terms of us safely applying AI to solve problems?
Ashley: It's a really important question. My answer is going to center around uncertainty and dealing with that in the context of the operation of the system, and let's say the implementation or the execution of the ethics of the system as well. But first, backing up to Francesca's comment, I just want to emphasize this notion of teaming and really embrace this narrative in my remarks here.
I've heard it said before that every machine is part of some human workflow. I think a colleague Matt Johnson at the Florida Institute for Human and Machine Cognition says that, which I really like. And so, just to make clear, whether we're talking about the cognitive enhancements, an application of AI where maybe you're doing information retrieval, or even a space exploration example, it's always part of a human-machine team. In the space exploration example, the scientists and the engineers are on the earth, maybe many light hours away, but the machines are helping them do science. But at the end of the day, the scientific discovery is really happening on earth with the scientists. And so, whether it's a machine operating remotely or by cognitive assistance, it's always part of a human-machine team. That's just something I wanted to amplify that Francesca said.
But coming back to the gaps, a lot of times I think what we're missing in our conversations is getting some structure around the role of uncertainty in these agents that we're trying to create that are going to help achieve that bright future that Francesca was referring to. To help us think about this at APL, we think about agents as needing to perceive, decide, act in teams. This is a framework that just helps us understand these general capabilities that we'll need and to start thinking about the role of uncertainty, and then combinations of learning and reasoning that would help agents to deal with that. And so, if you think about an agent pursuing goals, the first thing it has to do is get an understanding of the world states. This is this task of perception.
We often talk about, well, if an agent sees this or that, or if an agent finds itself in this situation, we want it to behave this way. Obviously, the trolley problem is an example we revisit often. I won't go into the details there, but the question is, I think, given some imperfect observation of the world, how does the structure of that uncertainty factor into the correct functioning of the agent in that situation? And then, how does that factor into the ethical, I'll say, choices or data-driven responses that an agent might have to that situation?
Then we talk about decision making. An agent has goals. In order to act on its goals, it has to decide about how certain sequences of actions would affect future states of the world. And then again how, in the context of an uncertain world, is the agent going to go about accurately evaluating possible future actions when it's outside of a gaming environment, for example. How does uncertainty play into that and its evaluation of possible actions? And then in the carrying out of those actions, there may be physical reasoning, geometric reasoning that has to happen. For example, if an agent is going to act in a physical space, or reasoning about a cyber-physical environment where there's critical infrastructure that needs to be protected or something like that.
And then finally, to Francesca's point, the interactions, or the teaming with other agents that may be teammates or actually may be adversarial. And so, how does the reasoning about what my teammates might be intending to do, what the state of my teammates might be in terms of cognitive load if it's a human teammate, what might the intent of adversarial agents be in confounding or interfering with the goals of the human-machine team?
And so, to recap a little bit, I think this notion of machines dealing with uncertainty in real world situations is one of the key challenges that we need to deal with over the coming decades. And so, I think having more explicit conversations about how uncertainty manifests in these situations, how you deal with it in the context of the real world operation of an AI-enabled system, and then how we give structure to the uncertainty in a way that should inform our ethical reasoning about the operation of these systems. I think that's a very worthy area of focus for us over the coming decades.
Ariel: Could you walk us through a specific example of how an AI system might be applied and what sort of uncertainties it might come across?
Ashley: Yeah, sure. So think about the situation where there's a dangerous environment, let's say, in a policing action or in a terrorist situation. Hey, there might be hostiles in this building, and right now a human being might have to go into that building to investigate it. We'll send a team of robots in there to do the investigation of the building to see if it's safe, and you can think about that situation as analogous for a number of possible different situations.
And now, let's think about the state of computer vision technology, where straight pattern recognition is hopefully a fair characterization of the state of the art, where we know we can very accurately recognize objects from a given universe of objects in a computer vision feed, for example. Well, now what happens if these agents encounter objects from outside of that universe of training classes? How can we start to bound the performance of the computer vision algorithm with respect to objects from unknown classes? You can start to get a sense from that progression, just from the perception part of that problem, from recognize, of these 200 possible objects, tell me which class it comes from, to having to do vision type tasks in environments that would present many new and novel objects that they may have to perceive and reason about.
You can think about that perception task now as extending to agents that might be in that environment and trying to ascertain from partial observations of what the agents might look like, partial observations of the things they might be doing to try to have some assessment of this is a friendly agent or this is an unfriendly agent, to reasoning about affordances of objects in the environment that might present our systems with ways of dealing with those agents that conform to ethical principles.
That was not a very, very concrete example, but hopefully starts to get one level deeper into the kinds of situations we want to put systems into and the kinds of uncertainty that might arise.
Francesca: To tie to what Ashley just said, we definitely need a lot more ways to have realistic simulations of what can happen in real life. So testbeds, sandboxes, that is definitely needed. But related to that, there is also this ongoing effort — which has already resulted in tools and mechanisms, but many people are still working on it — which is to understand better the error landscape that the machine learning approach may have. We know machine learning always has a small percentage of error in any given situation and that's okay, but we need to understand what's the robustness of the system in terms of that error, and also we need to understand the structure of that error space because this information can inform us on what are the most appropriate or less appropriate use cases for the system.
Of course, going from there, this understanding of the error landscape is just one aspect of the need for transparency on the capabilities and limitations of the AI systems when they are deployed. It’s a challenge that spans from academia or research centers to, of course, the business units and the companies developing and delivering AI systems. So that's why at IBM we are working a lot on this issue of collecting information during the development and the design phase around the properties of the systems, because we think that understanding of this property is very important to really understand what should or should not be done with the system.
And then, of course, there is, as you know, a lot of work around understanding other properties of the system. Like, fairness is one of the values that we may want to inject, but of course it's not as simple as it looks because there are many, many definitions of fairness and each one is more appropriate or less appropriate in certain scenarios and certain tasks. It is important to identify the right one at the beginning of the design and the development process, and then to inject mechanisms to detect and mitigate bias according to that notion of fairness that we have decided is the correct one for that product.
And so, this brings us also to the other big challenge, which is to help developers understand how to define these notions, these values like fairness that they need to use in developing the system — how to define them not just by themselves within the tech company, but also communicating with the communities that are going to be impacted by these AI product, and that may have something to say on what is the right definition of fairness that they care about. That's why, for example, another thing that we did, besides developing research and also products, but we also invest a lot in educating developers in trying to help them understand in their everyday jobs how to think about these issues, whether it's fairness, robustness, transparency, and so on.
And so, we built this very small booklet — we call it the Everyday AI Ethics Guide for Designers and Developers — that raises a lot of questions that should be in their mind in their everyday job. Because as you know, for example, if you don't think about bias or fairness during these development phases and you just check whether your product is fair or not or when it's ready to be deployed, then you may discover that actually you need to start from scratch again if you discover that it doesn't have the right notion of fairness.
Another effort that we really care a lot about in this effort to build teams of humans and machines is the issue of explainability, to make sure that it is possible to understand why these systems are recommending certain decisions. Explainability is something that, especially in this environment of teaming AI machines, is very important, because without this capability of AI systems of explaining why they are recommending certain decision, then the human being part of the team will not in the long run trust the AI system, so will not adopt it possibly. And so we will also lose the positive and beneficial effect of the AI system.
The last thing that I want to say is that this education of developers extends actually much beyond the developers to also the policy makers. That's why it's important to have a lot of interaction with policy makers that need to really be educated about the state of the art, about the challenges, about the limits of current AI, in order to understand how to best drive the current technology, to be more and more advanced, but also beneficial and driven towards the beneficial efforts. And what are the right mechanisms to drive the technology into the direction that we want? Still needs a lot more multi-stakeholder discussion to really achieve the best results, I think.
Ashley: Just picking up on a couple of those themes that Francesca raised: first, I just want to touch on simulations. At the applied physics laboratory, one of the core things we do is develop systems for the real world. And so, as the tools of artificial intelligence are evolving, the art and the science of systems engineering is starting to morph into this AI systems engineering regime. And we see simulation as key, more key than it's ever been, into developing real world systems that are enabled by AI.
One of the things we're really looking into now is what we call live virtual constructive simulations. These are simulations that you can do distributed learning for agents in a constructive mode where you have highly parallelized learning, but where you actually have links and hooks for live interactions with humans to get the human-machine teaming. And then finally, bridging the gap between simulation and real world where some of the agents represented in the context of the human-machine teaming functionality can be virtual and some can actually be represented by real systems in the real world. And so, we think that these kinds of environments, these live virtual constructive environments, will be important for bridging the gap from simulation to real.
Now, in the context of that is this notion of sharing information. If you think about the complexity of the systems that we're building, and the complexity and the uncertainty of the real world conditions — whether that's physical or cyber or what have you — it's going to be more and more challenging for a single development team to analytically characterize the performance of the system in the context of real-world environment. And so, I think as a community we're really doing science; We're performing science, fielding these complex systems in these real-world environments. And so, the more we can make that a collective scientific exploration where we're setting hypotheses, performing these experiments — these experiments of deploying AI in real world situations — the more quickly we'll make progress.
And then, finally, I just wanted to talk about accountability, which I think builds on this notion of transparency and explainability. From what I can see — and this is something we don't talk about enough, I think — is I think we need to change our notion of accountability when it comes to AI-enabled systems. I think our human nature is we want individual accountability for individual decisions and individual actions. If an accident happens, our whole legal system, our whole accountability framework is, "Well, tell me exactly what happened that time," and I want to get some accountability based on that and I want to see something improve based on that. Whether it's a plane crash or a car crash, or let's say there's corruption in a Fortune 500 company — we want see the CFO fired and we want to see a new person hired.
I think when you look at these algorithms, they're driven by statistics, and the statistics that drive these models are really not well suited for individual accountability. It's very hard to establish the validity of a particular answer or classification or something that comes out of the algorithm. Rather, we're really starting to look at the performance of these algorithms over a period of time. It's hard to say, "Okay, this AI-enabled system: tell me what happened on Wednesday," or, "Let me hold you accountable for what happened on Wednesday." And more so, "Let me hold you accountable for everything that you did during the month of April that resulted in this performance."
And so, I think our notion of accountability is going to have to embrace this notion of ensemble validity, validity over a collection of activities, actions, decisions. Because right now, I think if you look at the underlying mathematical frameworks for these algorithms, they're not well supported for this notion of individual accountability for decisions.
Francesca: Accountability is very important. It needs a lot more discussion. This is one of the topic also that we have been discussing in this initiative by the European Commission in defining the AI Ethics Guidelines for Europe, and accountability is one of the seven requirements. But it's not easy to define what it means. What Ashley said is one possibility: Change our idea of accountability from one specific instance to over several instances. That's one possibility, but I think that that's something that needs a lot more discussion with several stakeholders.
Ariel: You've both mentioned some things that sound like we're starting to move in the right direction. Francesca, you talked about getting developers to think about some of the issues like fairness and bias before they start to develop things. You talked about trying to get policy makers more involved. Ashley, you mentioned the live virtual simulations. Looking at where we are today, what are some of the things that you think have been most successful in moving towards a world where we're considering AI safety more regularly, or completely regularly?
Francesca: First of all, we've gone a really long way in a relatively short period of time, and the Future of Life Institute has been instrumental in building the community, and everybody understands that the only approach to address this issue is a multidisciplinary, multi-stakeholder approach. The Future of Life Institute, with the first Puerto Rico conference, showed very clearly that this is the approach to follow. So I think that in terms of building the community that discusses and identifies the issues, I think we have done a lot.
I think that at this point, what we need is greater coordination and also redundancy removal among all these different initiatives. I think we have to find, as a community, the main issues and the main principles and guidelines that we think are needed for the development of more advanced forms of AI, starting from the current state of the art. If you look at the values, at these guidelines or lists of principles around AI ethics from the values initiatives, they are of course different from each other but they have a lot in common. So we really were able to identify these issues, and this identification of the main issues is important as we move forward to more advanced versions of AI.
And then, I think another thing that also we are doing in a rather successful though not complete way is trying to move from research to practice. From high level principles to concrete, develop, and deploy the products that can embed these principles and guidelines into not just the scientific papers that are published, but also into the platform, the services, and the tool kits that companies use with their clients. We needed an initial phase where there were high level discussions about guidelines and principles, but now we are in the second phase where these go and percolate down to the business units and to how products are built and deployed.
Ashley: Yeah, just building on some of Francesca's comments, I've been very inspired by the work of the Future of Life Institute and the burgeoning, I'll say, emerging AI safety community. Similar to Francesca's comment, I think that the real frontier here is now taking a lot of that energy, a lot of that academic exploration, research, and analysis and starting to find the intersections of a lot of those explorations with the real systems that we're building.
You're definitely seeing within IBM, as Francesca mentioned, within Microsoft, within more applied R & D organizations like Johns Hopkins APL, where I am, internal efforts to try to bridge the gap. And what I really want to try to work to catalyze in the coming years is a broader, more community-wide intersection between the academic research community looking out over the coming centuries and the applied research community that's looking out over the coming decades, and find the intersection there. How do we start to pose a lot of these longer term challenge problems in the context of real systems that we're developing?
And maybe we get to examples. Let's say, for ethics, beyond the trolley problem and into posing problems that are more real-world or closer, better analogies to the kinds of systems we're developing, the kinds of situations they will find themselves in, and start to give structure to some of the underlying uncertainty. Having our debates informed by those things.
Ariel: I think that transitions really nicely to the next question I want to ask you both, and that is, over the next 5 to 10 years, what do you want to see out of the AI community that you think will be most useful in implementing safety and ethics?
Ashley: I'll probably sound repetitive, but I really think focusing in on characterizing — I think I like the way Francesca put it — the error landscape of a system as a function of the complex internal states and workings of the system, and the complex and uncertain real-world environments, whether cyber or physical that the system will be operating in, and really get deeper there. It's probably clear to anyone that works in the space that we really need to fundamentally advance the science and the technology. I'll start to introduce the word now: trust, as it pertains to AI-enabled systems operating in these complex and uncertain environments. And again, starting to better ground some of our longer-term thinking about AI being beneficial for humanity and grounding those conversations into the realities of the technologies as they stand today and as we hope to develop and advance them over the next few decades.
Francesca: Trust means building trust in the technology itself — and so the things that we already mentioned like making sure that it's fair, value aligned, robust, explainable — but also building trust in those that produce the technology. But then, I mean, this is the current topic: How do we build trust? Because without trust we're not going to adopt the full potential of the beneficial effect of the technology. It makes sense to also think in parallel, and more in the long-term, what's the right governance? What's the right coordination of initiatives around AI and AI ethics? And this is already a discussion that is taking place.
And then, after governance and coordination, it's also important with more and more advanced versions of AI, to think about our identity, to think about the control issues, to think in general about this vision of the future, the wellbeing of the people, of the society, of the planet. And how to reverse engineer, in some sense, from a vision of the future to what it means in terms of a behavior of the technology, behavior of those that produce the technology, and behavior of those that regulate the technology, and so on.
We need a lot more of this reverse engineering approach, where instead of starting from the current state of the art of the technology and saying, "Okay, these are the properties that I think I want in this technology: fairness, robustness, transparency, and so on, because otherwise I don't like this technology to be deployed without these properties." And then see what happens in the next version, more advanced version of the technology, and think about possibly new properties and so on. This is one approach, but the other approach is that, "Okay, this is the vision of life in, I don't know, 50 years from now. How do I go from that to the kind of the technology, to the direction that I want to push the technology towards to achieve that vision?
Ariel: We are getting a little bit short on time, and I did want to follow up with Ashley about his other job. Basically, Ashley, as far as my understanding, you essentially have a side job as a hip hop artist. I think it would be fun to just talk a little bit in the last couple of minutes that we have about how both you and Francesca see artificial intelligence impacting these more creative fields. Is this something that you see as enhancing artists' abilities to do more? Do you think there's a reason for artists to be concerned that AI will soon be a competition for them? What are your thoughts for the future of creativity and AI?
Ashley: Yeah. It's interesting. As you point out, over the last decade or so, in addition to furthering my career as an engineer, I've also been a hip hop artist and I've toured around the world and put out some albums.I think where we see the biggest impact of technology on music and creativity, I think, is, one, in the democratization of access to creation. Technology is a lot cheaper. Having a microphone and a recording setup or something like that, from the standpoint of somebody that does vocals like me, is much more accessible to many more people. And then, you see advances and — you know, when I started doing music I would print CDs and press vinyl. There was no iTunes. And just, iTunes has revolutionized how music is accessed by people, and more generally how creative products are accessed by people in streaming, etc. So I think looking backward, we've seen most of the impact of technology on those two things: access to the creation and then access to the content.
Looking forward, will those continue to be the dominant factors in terms of how technology is influencing the creation of music, for example? Or will there be something more? Will AI start to become more of a creative partner? We'll see that and it will be evolutionary. I think we already see technology being a creative partner more and more so over time. A lot of the things that I studied in school — digital signal processing, frequency, selective filtering — a lot of those things are baked into the tools already. And just as we see AI helping to interpret other kinds of signal processing products like radiology scans, we'll see more and more of that in the creation of music where an AI assistant — for example, if I'm looking for samples from other music — an AI assistant that can comb through a large library of music and find good samples for me. Just as we do with Instagram filters — an AI suggesting good filters for pictures I take on my iPhone — you can see in music AI suggesting good audio filters or good mastering settings or something, given a song that I'm trying to produce or goals that I have for the feel and tone of the product.
And so, already I think as an evolutionary step, not even a revolutionary step, AI becoming more present in the creation of music. I think maybe, as in other application areas, we may see, again, AI being more of a teammate, not only in the creation of the music, but in the playing of the music. I heard an article or a cast on NPR about a piano player that developed an AI accompaniment for himself. And so, as he played in a live show, for example, there would be an AI accompaniment and you could dial back the settings on it in terms of how aggressive it was in rhythm and time, where it situated with respect to the lead performer. Maybe in hip hop we'll see AI hype men or AI DJs. It's expensive to travel overseas, and so somebody like me goes overseas to do a show, and instead of bringing a DJ with me, I have an AI program that can select my tracks and add cuts at the right places and things like that. So that was a long-winded answer, but there's a lot there. Hopefully that was addressing your question.
Ariel: Yeah, absolutely. Francesca, did you have anything you wanted to add about what you think AI can do for creativity?
Francesca: Yeah. I mean, of course I'm less familiar of what AI is already doing right now, but I am aware of many systems from companies into the space of delivering content or music or so on, systems where the AI part is helping humans develop their own creativity even farther. And as Ashley said, I mean, I hope that in the future AI can help us be more creative — even people that maybe are less able than Ashley to be creative themselves. And I hope that this will enhance the creativity of everybody, because this will enhance the creativity, yes, in hip hop or in making songs or in other things, but also I think it will help to solve some very fundamental problems because having a population which is more creative, of course, is more creative in everything.
So in general, I hope that AI will help us human beings be more creative in all aspects of our life besides entertainment — which is of course very, very important for all of us for the wellbeing and so on — but also in all the other aspects of our life. And this is the goal that I think — going to the beginning where I said AI's purpose should be the one of enhancing our own capabilities. And of course, creativity is also a very important capability that human beings have.
Ariel: Alright. Well, thank you both so much for joining us today. I really enjoyed the conversation.
Francesca: Thank you.
Ashley: Thanks for having me. I really enjoyed it.
Ariel: For all of our listeners, if you have been enjoying this podcast, please take a moment to like it or share it and maybe even give us a good review. And we will be back again next month.